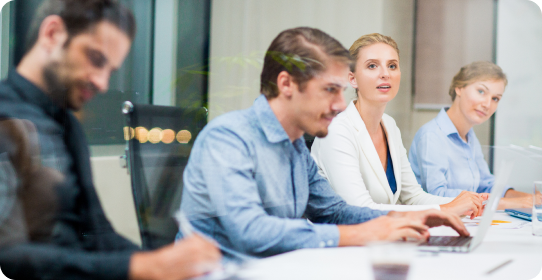
In today's fast-paced business landscape, staying ahead of the competition requires efficient and effective solutions. According to Microsoft’s Work Trend Index, nearly 70% of employee report that they don’t have sufficient time in the day to focus on “work”, with more time being spent Communicating than Creating.
Microsoft 365 Copilot is designed, with Microsoft’s cloud trust platform at its core, to allow for employees to both be more productive, reduce the time spent searching for information, performing mundane tasks, and other low-value activities.
The process of loading data into a traditional data warehouse typically follows the pattern of Extracting, Transforming, and Loading (ETL). Most businesses use a dedicated server to perform these operations.
However, with MPP architecture and the ability to load data fast, the new standard is becoming Extract, Load, and Transform or ELT.
This approach allows for the original dataset to be loaded into the data warehouse with minimal modifications and later transformed within the data warehouse.
Data Import to Azure SQL Data Warehouse
Many tools are available to facilitate the loading of data into Azure SQL Data Warehouse from a variety of sources.
Some of the tools are native to the Microsoft Azure and SQL Server eco-system:
- PolyBase
- Azure Data Factory
- SQL Server Integration ServicesAZCopy
- bcp
Others are provided by third party software vendors (e.g. Data Platform Studio by Redgate). In addition, Azure Stream Analytics can be used to load streaming data into Azure SQL Data Warehouse in near-real-time.
Data Import Tools
Polybase
PolyBase uses extensions to the widely-used Transact-SQL (T-SQL) language to load data from Azure Blob storage, HDInsight, or Azure Data Lake Store.
Azure Blob storage limits file sizes to 1 TB, but using Azure Data Lake Store removes such restrictions and allows unlimited file sizes or number of files stored.
PolyBase provides a fast and efficient method of loading data into Azure SQL Data Warehouse that takes full advantage of the Data Warehouse’s MPP architecture.
Azure Data Factory
Azure Data Factory is used to orchestrate data movement between various types of data repositories located on-premises or in Azure to Azure SQL Data Warehouse.
Azure Data Factory pipelines provide powerful capabilities for defining, scheduling and monitoring the loading of your data to Azure SQL Data Warehouse (or to other destinations).
Azure Machine Learning can perform complex processing while loading data. For example, Azure Data Factory can integrate with Azure Machine Learning models to make predictions and write the results to Azure SQL Data Warehouse.
Azure Data Factory pipelines can be authored using wizard-like interfaces in Azure Portal or using Visual Studio.
AZCopy
AZCopy is a command-line utility can be used to load data from flat files to Azure Blob Storage.
It is typically recommended for datasets up to 10 TB in size. AZCopy is frequently used in conjunction with the bcp utility and PolyBase (e.g. the bcp utility exports data from SQL Server databases into flat files, AZCopy loads the data into Azure blob storage, while PolyBase loads the data from blob storage into Azure SQL Data Warehouse).
bcp
bcp utility can also be used as a standalone tool to load data from flat files directly into the Azure SQL Data Warehouse. This approach is suitable for small data sets.
Data Platform Studio
Redgate's Data Platform Studio (DPS) is another powerful tool that can facilitate data import from SQL Server into the Azure Data Warehouse.
DPS compresses data during upload to Azure, which increases the efficiency of the transfer, and takes less time than a manual migration.
Signing in with an Azure Account will give DPS immediate access to relevant storage and Azure SQL Data Warehouse resources in your account, which provides for quick and convenient integration.
DPS ensures data security by encrypting data and passing it from the local network directly to your Azure resource and not through any additional third-party servers.
Azure Stream Analytics
In addition to the batch-oriented processes described above, Azure Stream Analytics is a fully managed complex event processing service that can be used to load massive volumes of streaming data into Azure SQL Data Warehouse in near-real-time.
In such a scenario:
- Data originates from a variety of event producers or IoT Devices
- Passes through an Azure Event Hub (or Azure IoT Hub)
- Is processed by an Azure Stream Analytics Job, and
- Is loaded directly into the Azure SQL Data Warehouse for subsequent analysis.
Furthermore, Azure Stream Analytics seamlessly integrates with Azure Machine Learning models to make predictions on incoming data in near real-time, while preserving the results in Azure SQL Data Warehouse.
Additional Resources
Best practices for Azure SQL Data Warehouse
Service Level Agreement (SLA) for SQL Data Warehouse
(source)
Want to learn more about PolyBase?
Check out our blog post:
"Analyzing Unstructured Data with PolyBase in SQL 2016."